Machine Learning for Drug Discovery: Accelerating the Path to New Therapies
In an industry where bringing a single drug to market traditionally costs billions of dollars and takes over a decade, artificial intelligence and machine learning have emerged as game-changing technologies in pharmaceutical research. These computational approaches are transforming how researchers identify novel drug targets, optimize candidate molecules, and design more efficient clinical trials—potentially saving years of development time and billions in costs.
The AI Revolution in Target Identification
Target identification—determining which proteins or biological pathways to focus on for therapeutic intervention—has historically been a bottleneck in drug discovery. Today, ML algorithms are rapidly accelerating this process by analyzing vast datasets to identify promising drug targets with unprecedented speed.
BenevolentAI demonstrated this power when its AI platform identified a promising target for amyotrophic lateral sclerosis (ALS), a complex neurodegenerative disorder with no current cure. By analyzing patient data, biological pathways, and protein interactions, the platform pinpointed a potential target that researchers had not previously considered.
Similarly, DeepDTnet—an AI system embedded with 15 variations of phenotypic, chemical, cellular profiles, and genomic data—successfully identified novel targets for existing FDA-approved drugs, helping bridge the translational void in drug development by revealing new uses for compounds that have already undergone safety testing.
Enhancing Molecular Design and Optimization
After identifying promising targets, researchers must design drug candidates that can effectively engage them. Graph Convolutional Networks (GCNs) have proven particularly effective for modeling molecular structures due to their ability to capture intricate relationships between atoms and bonds.
According to recent benchmarking studies, GCN-based models consistently outperform older computational methods in predicting molecular properties and bioactivity—with some reporting prediction accuracies exceeding 85% for complex tasks like blood-brain barrier penetration and toxicity assessment.
Machine learning approaches are now also capable of generating entirely new molecular structures. For example, researchers at Insilico Medicine utilized ML to design INS018_055, a new inhibitor of DDR1 kinase implicated in fibrosis. The entire process—from target selection to preclinical candidate nomination—took just 18 months compared to the typical 4-5 years using traditional methods.
Revolutionizing Clinical Trials
Clinical trials represent the most expensive and time-consuming phase of drug development, with failure rates exceeding 80% across all phases. Machine learning approaches are being deployed to address key challenges:
Patient Selection and Stratification
ML algorithms can identify patients most likely to respond to specific treatments based on biomarkers, genetic profiles, and other clinical characteristics. This targeted approach increases the likelihood of demonstrating efficacy while requiring fewer participants.
In the BATTLE clinical trial for lung cancer treatments, ML models were used to identify key parameters in drug sensitivity sites across tumors, improving the classification of responders versus non-responders.
Adaptive Trial Design
AI enables adaptive trial designs that modify specific parameters based on interim results. The I-SPY 2 breast cancer trial used adaptive randomization powered by AI algorithms to dynamically assign patients to treatment arms most likely to yield positive outcomes, reducing the time required to identify promising therapies.
Remote Patient Monitoring
In one of the most significant medtech AI trends of recent years, Remote Patient Monitoring (RPM) combined with AI is transforming how clinical trial data is collected. According to recent studies, RPM can cut hospital readmissions by up to 38% and reduce emergency room visits by up to 78%.
When Atrium Health created a Virtual Hospital in response to COVID-19, the system used RPM devices and AI algorithms to monitor patients remotely, allowing approximately two-thirds of COVID-positive patients to be managed at home, with only 3% requiring hospitalization.
Funding Trends: Unprecedented Investment
The financial trajectory of AI in drug discovery reflects growing confidence in these technologies. The global AI in Remote Patient Monitoring market was valued at $1.5 billion in 2023 and is forecast to grow at a CAGR of 27.5%, reaching $8.4 billion by 2030.
The broader AI in drug discovery market is similarly experiencing explosive growth, projected to increase from $3.5 billion in 2023 to $7.9 billion by 2030, with a CAGR of 23.72%.
In 2023 alone, AI drug discovery companies raised over $2 billion in investment capital, with major players including Exscientia, Recursion Pharmaceuticals, and Atomwise leading the way. Pharmaceutical giants are also heavily investing, with companies like Pfizer, Merck, and AstraZeneca establishing dedicated AI divisions and partnership programs.
Challenges and Future Directions
Despite remarkable progress, several challenges remain. The pharmaceutical industry continues to grapple with how to aggregate, normalize, and integrate disparate data sources while maintaining data privacy and security. Federated learning approaches that allow models to be trained across multiple institutions without sharing raw data are emerging as promising solutions.
The "black box" nature of some deep learning approaches raises concerns about interpretability and trustworthiness. As research in "explainable AI" advances, we're seeing increased focus on developing models that not only make accurate predictions but also provide insights into the reasoning behind those predictions.
Regulatory frameworks for AI-driven drug discovery are still evolving. The FDA and other regulatory bodies are working to establish guidelines for validating AI approaches while ensuring patient safety remains paramount.
Conclusion
Machine learning is fundamentally transforming drug discovery from a largely empirical, trial-and-error process to a more rational, data-driven approach. The unprecedented level of investment reflects growing confidence in the potential of these approaches to address some of the most significant challenges in drug development. For patients waiting for new treatments, these innovations hold tremendous promise for delivering therapies faster and more efficiently than ever before.
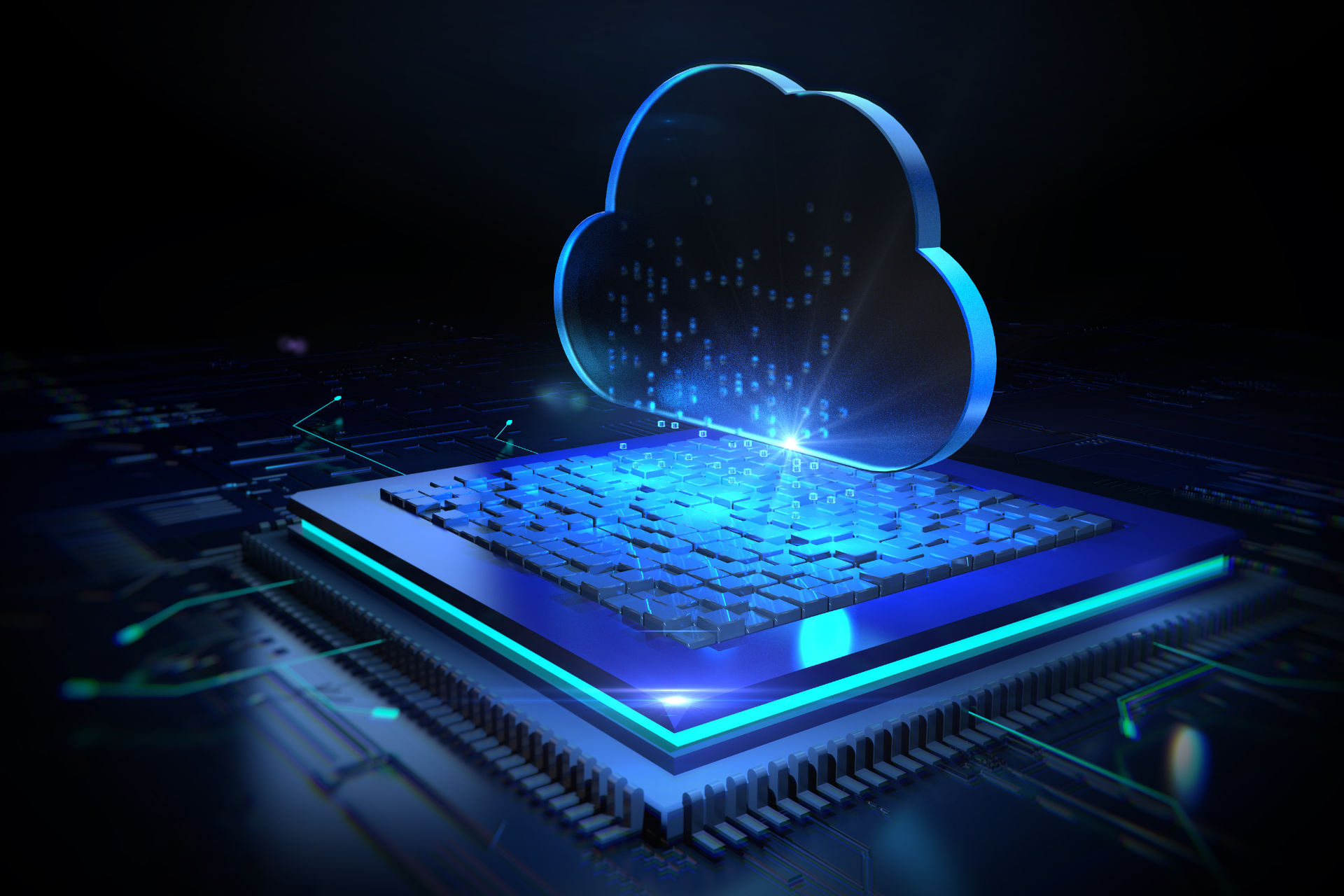
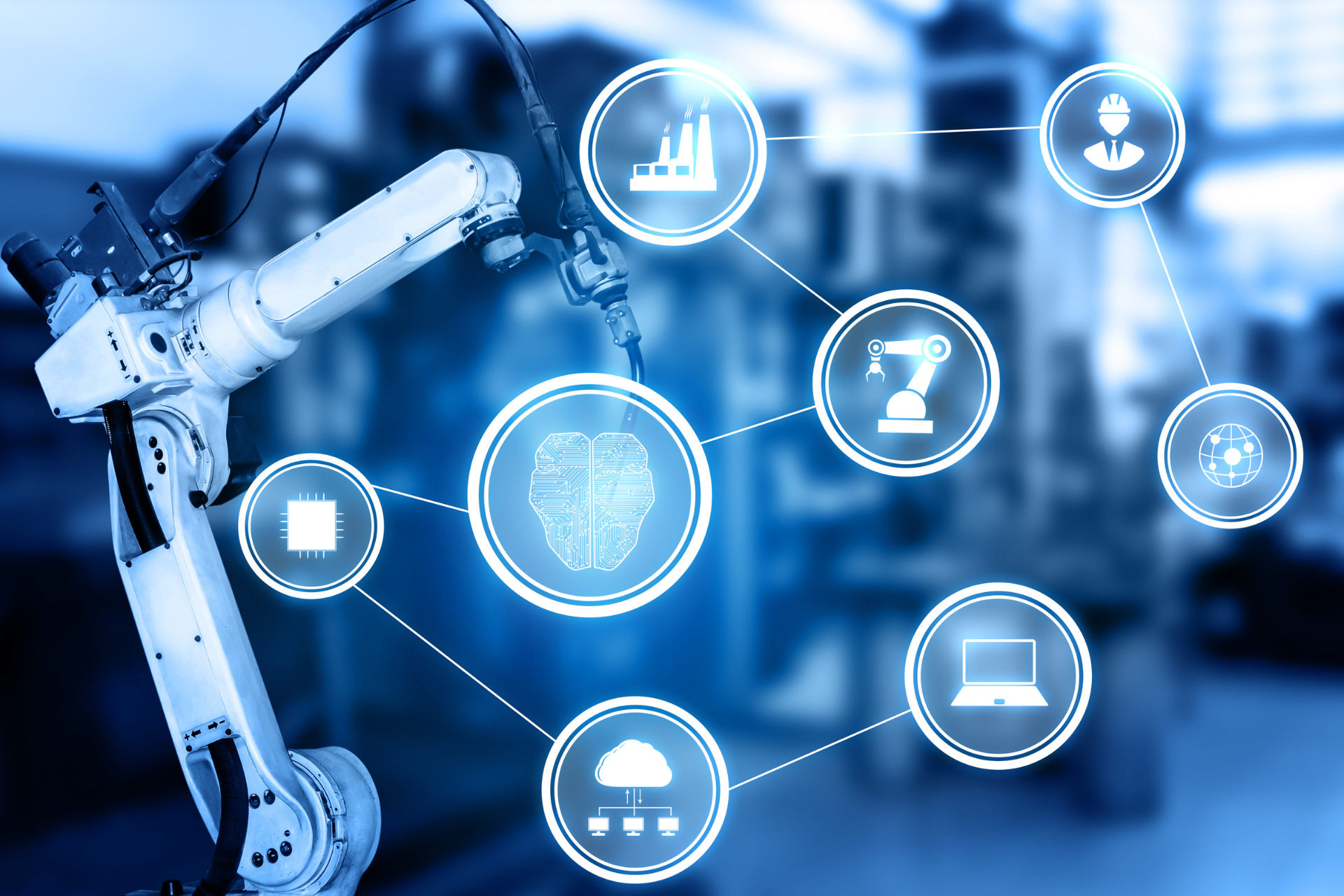
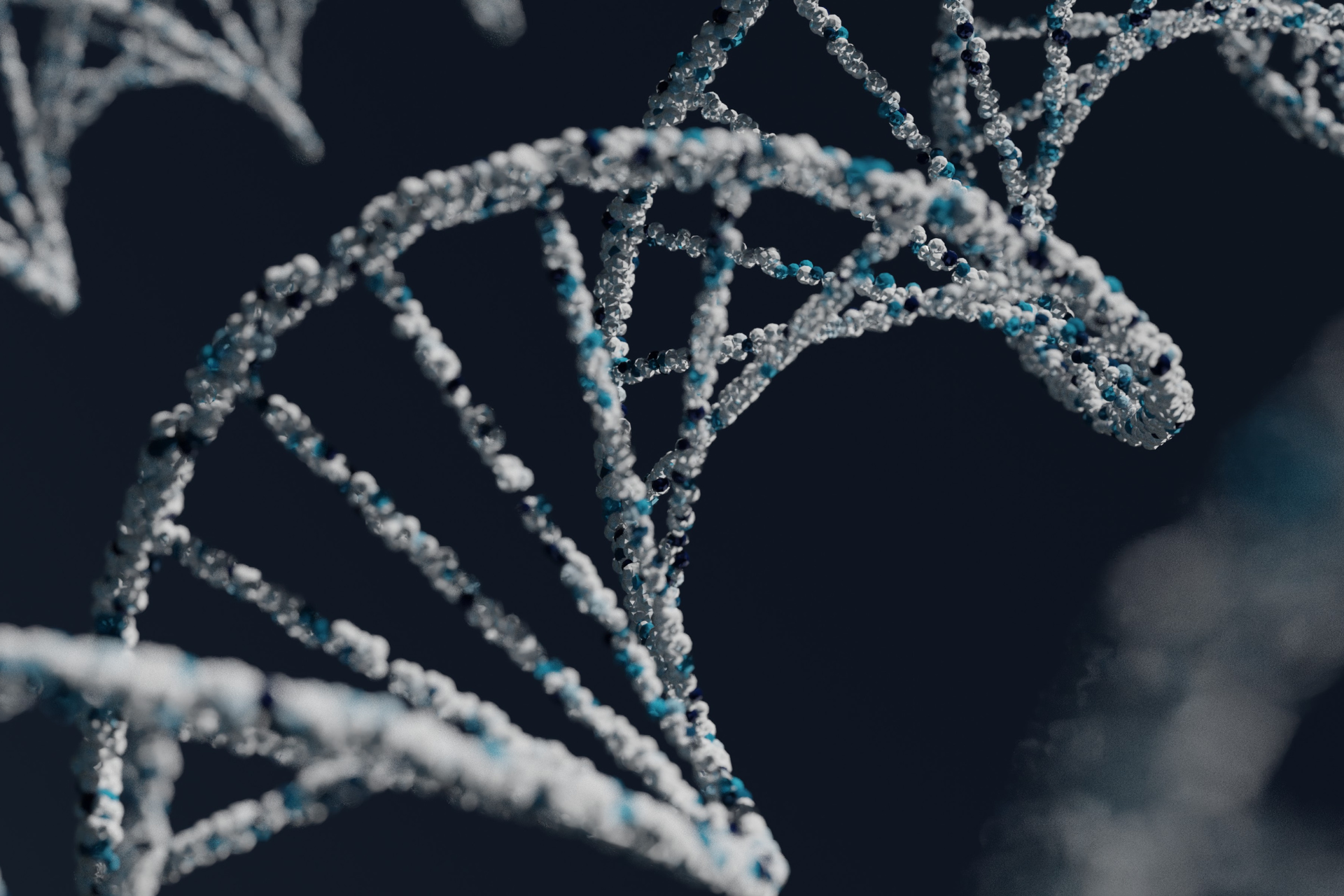
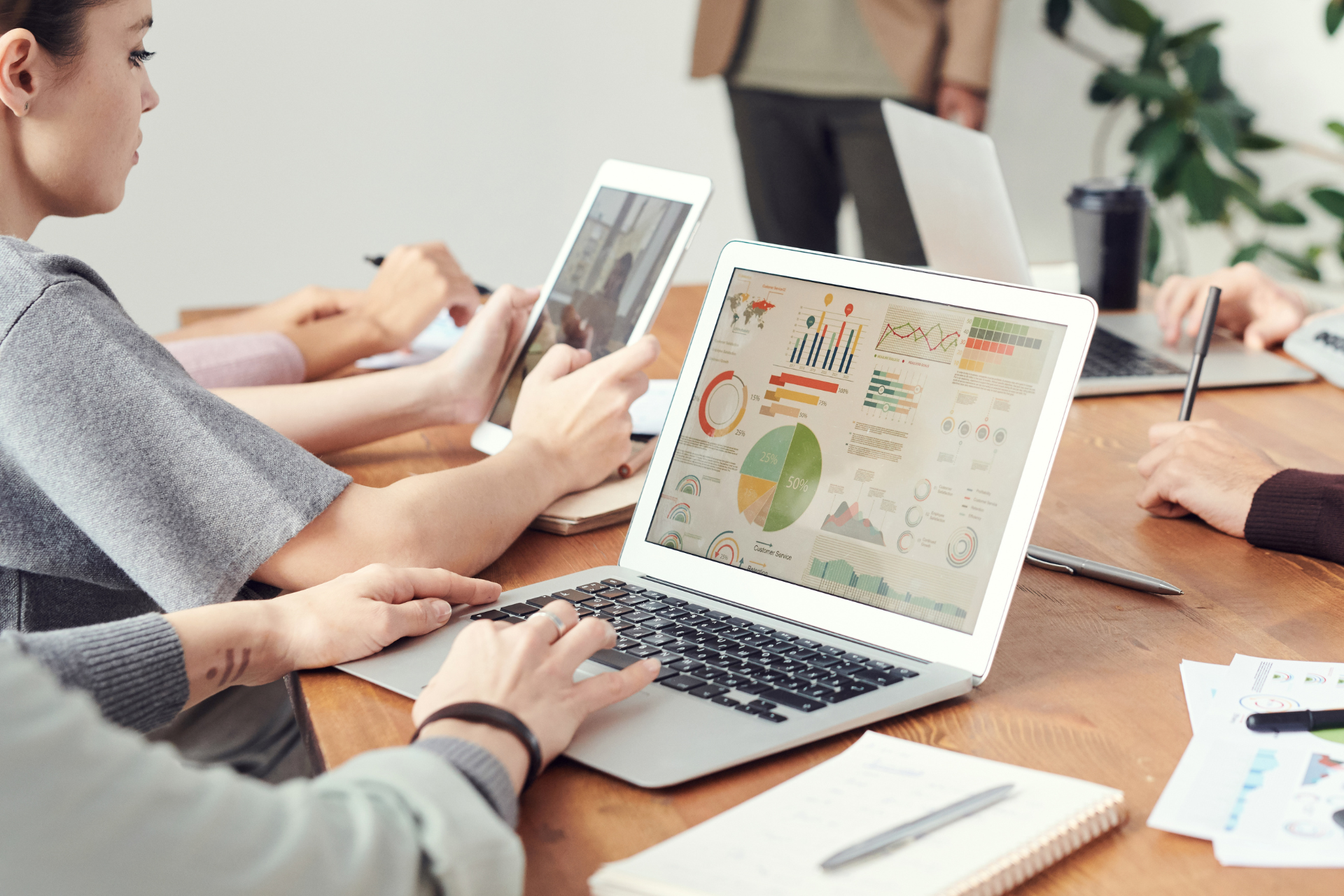
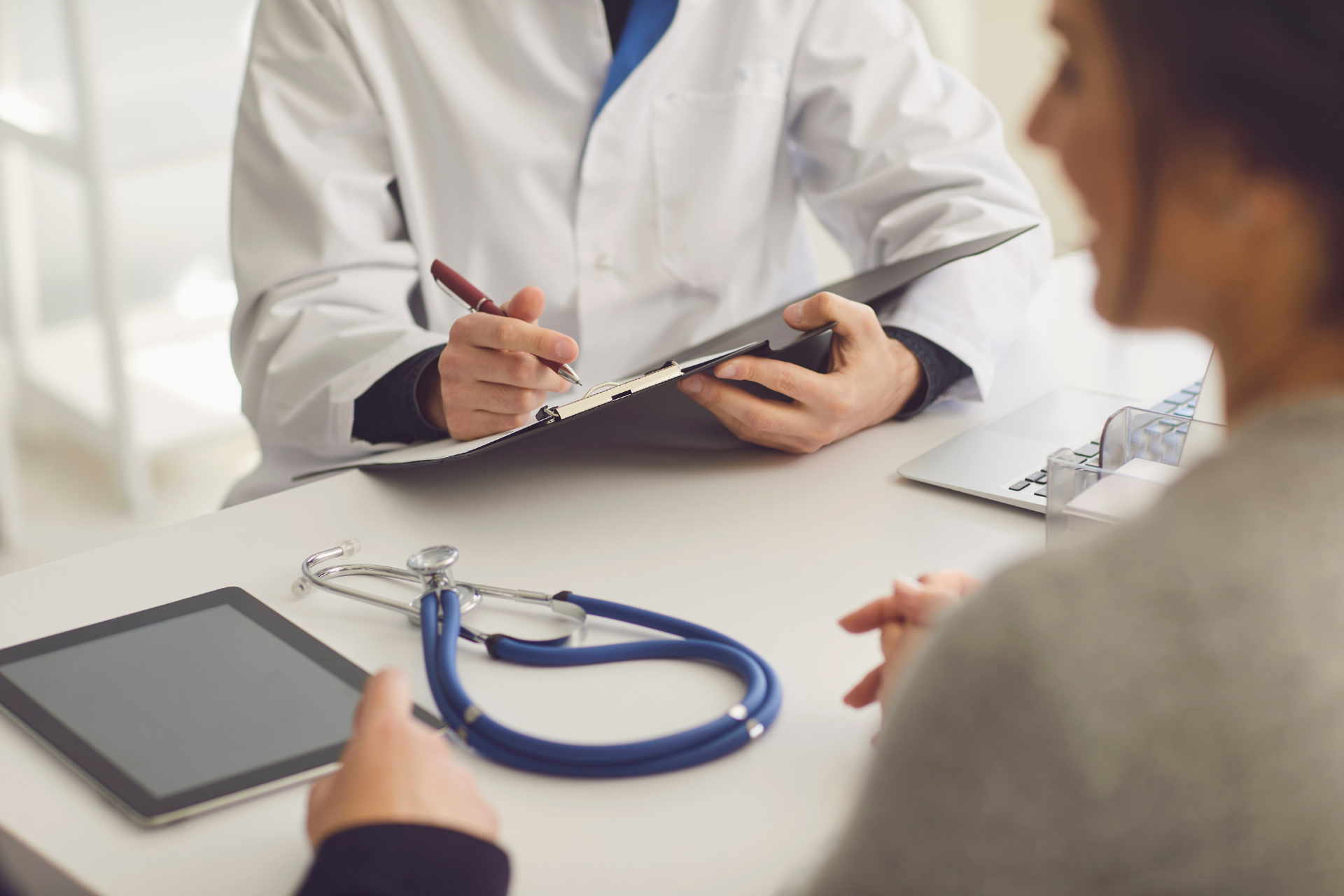
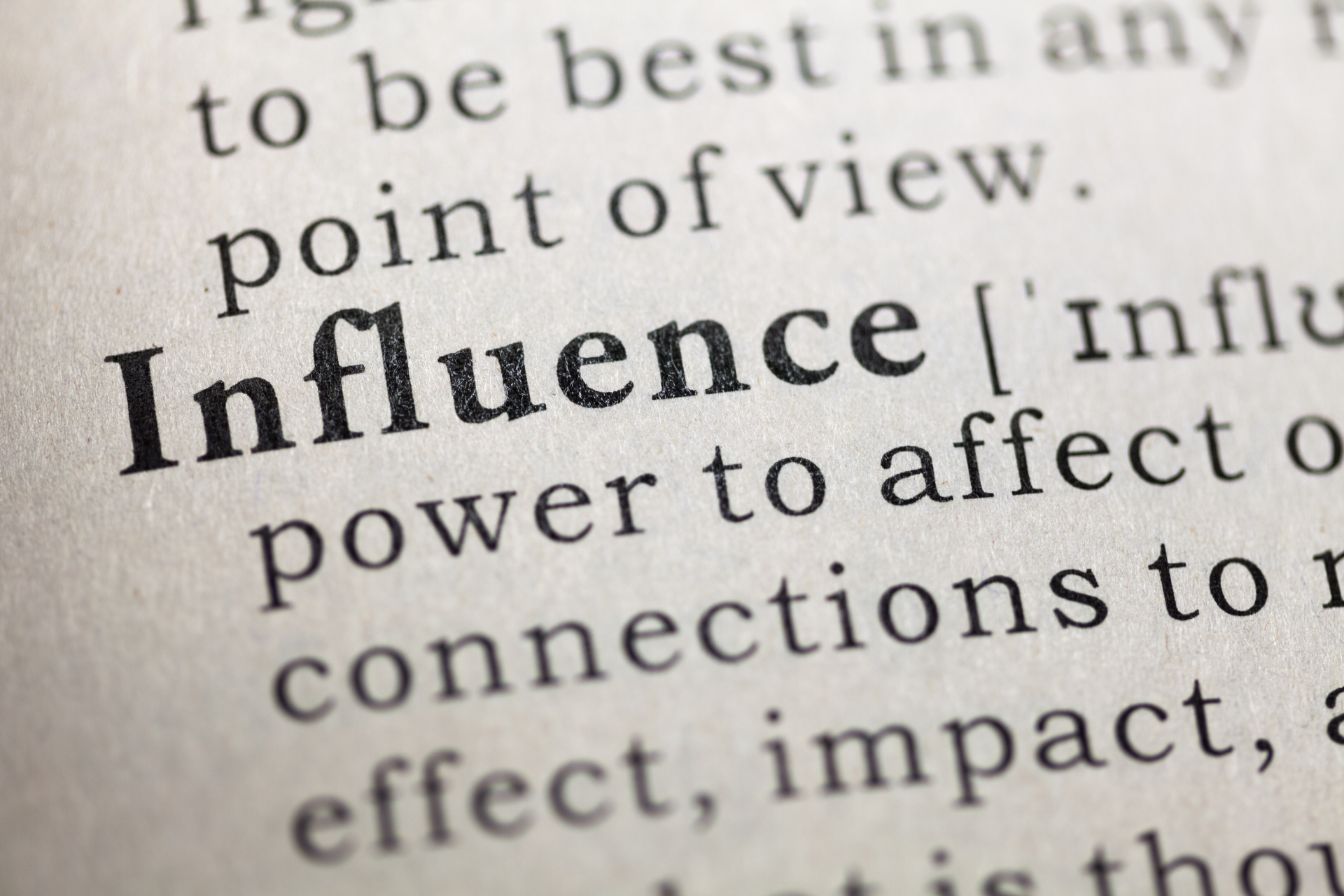
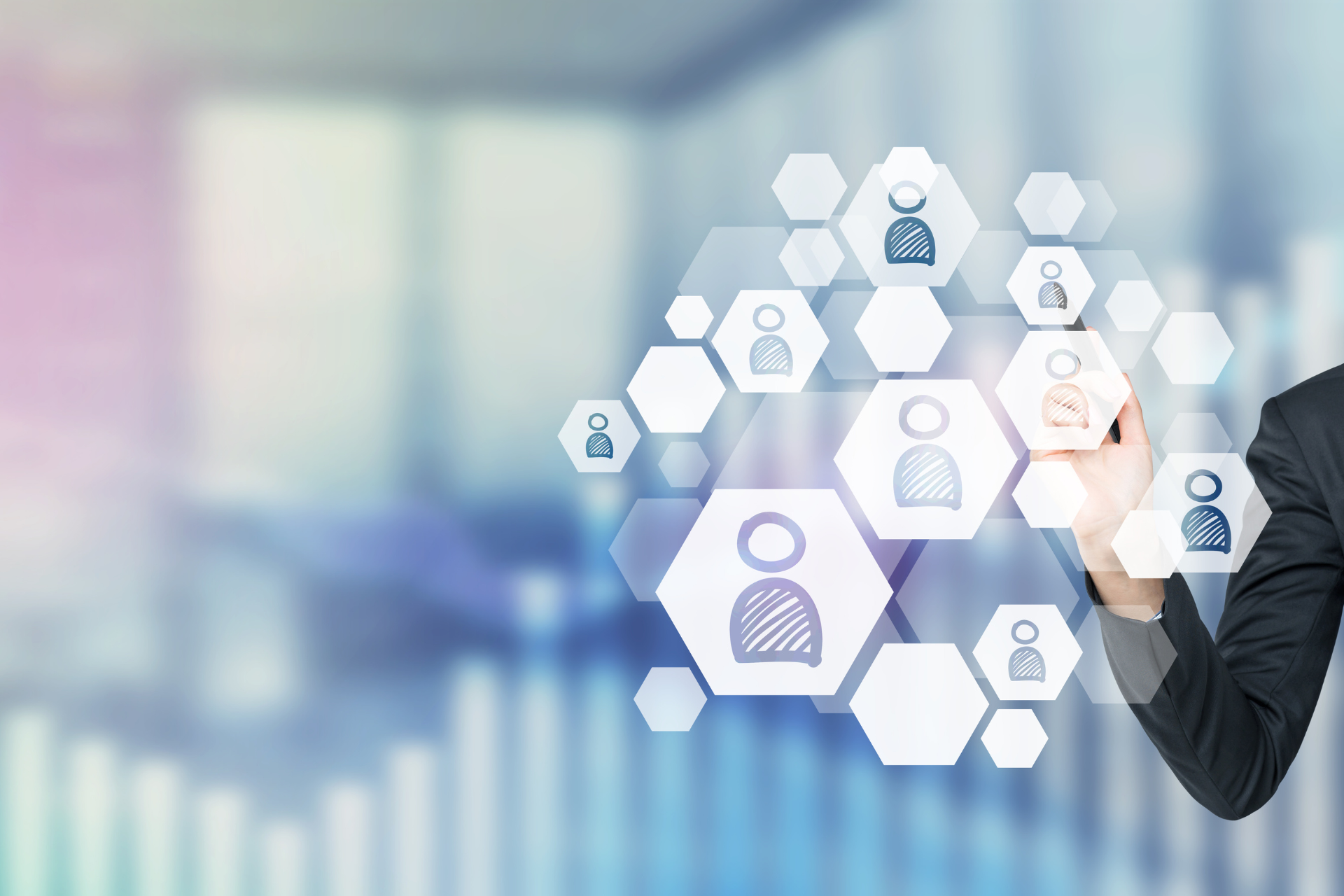
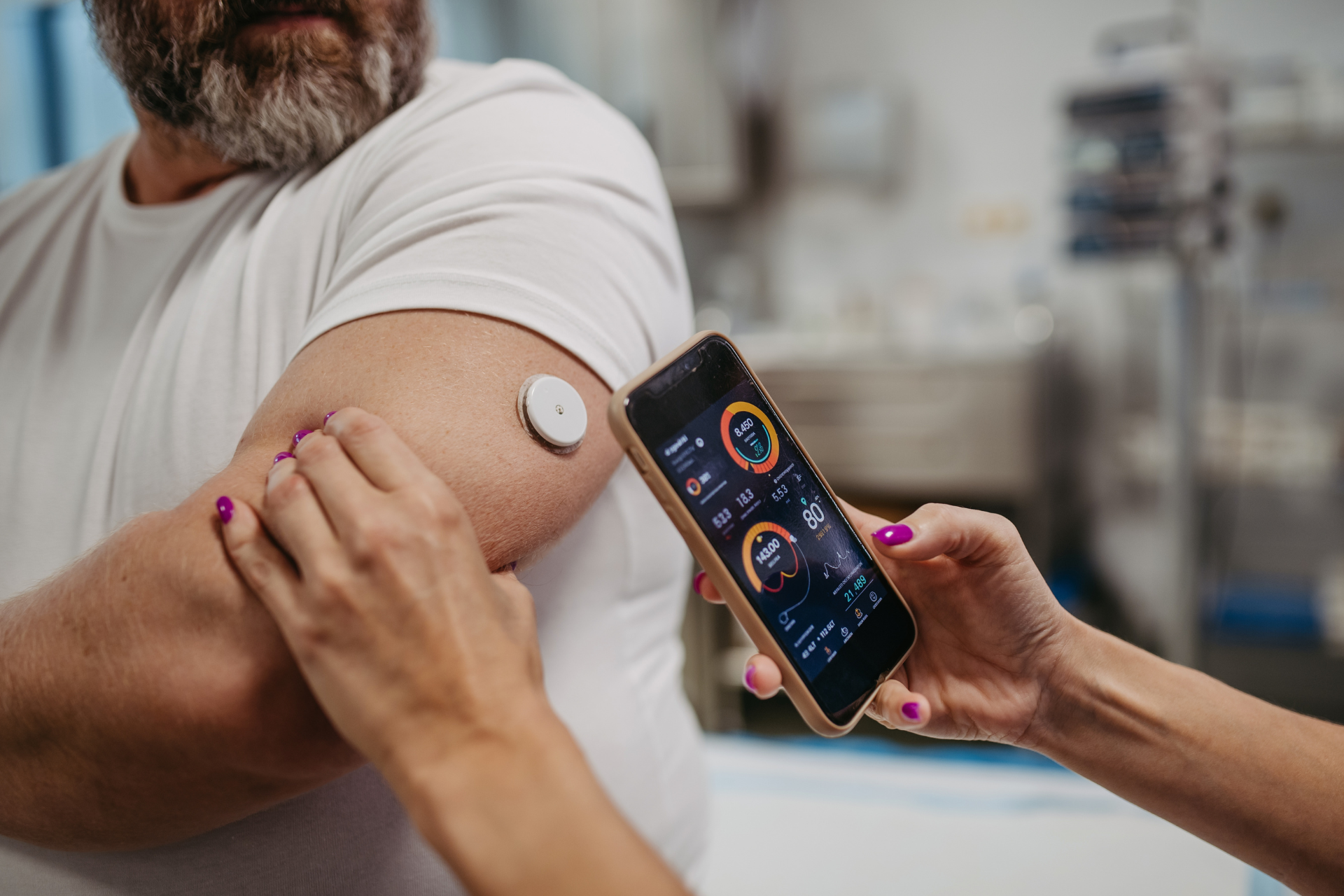